Resources
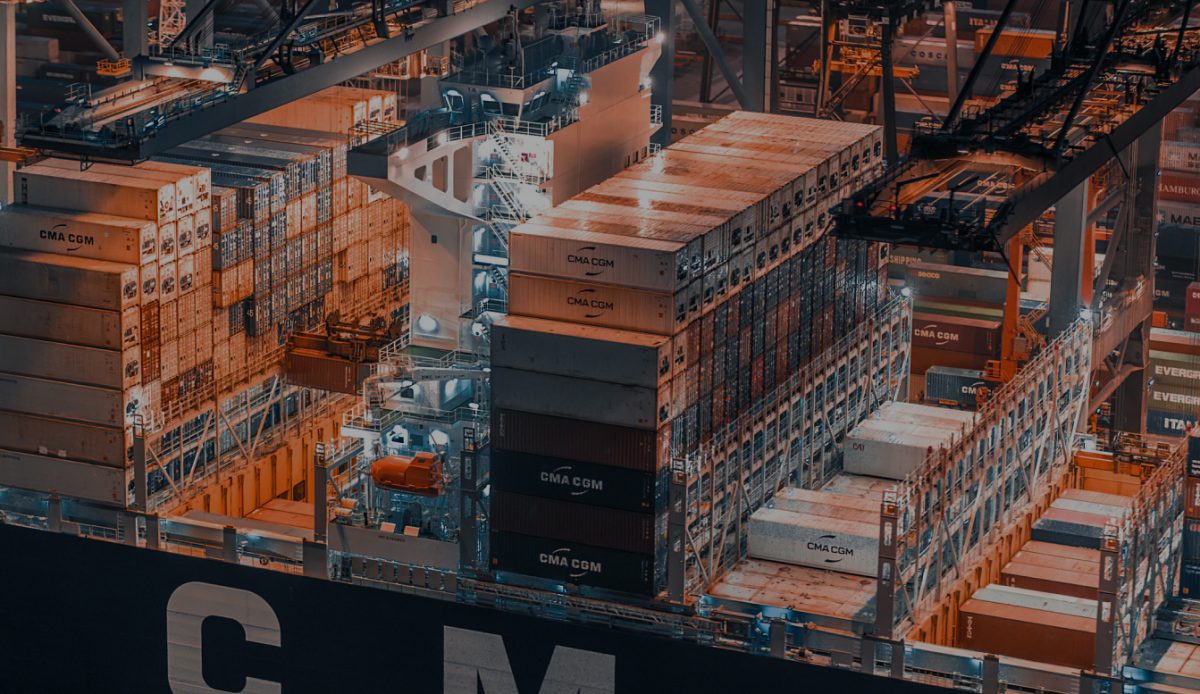
All resources
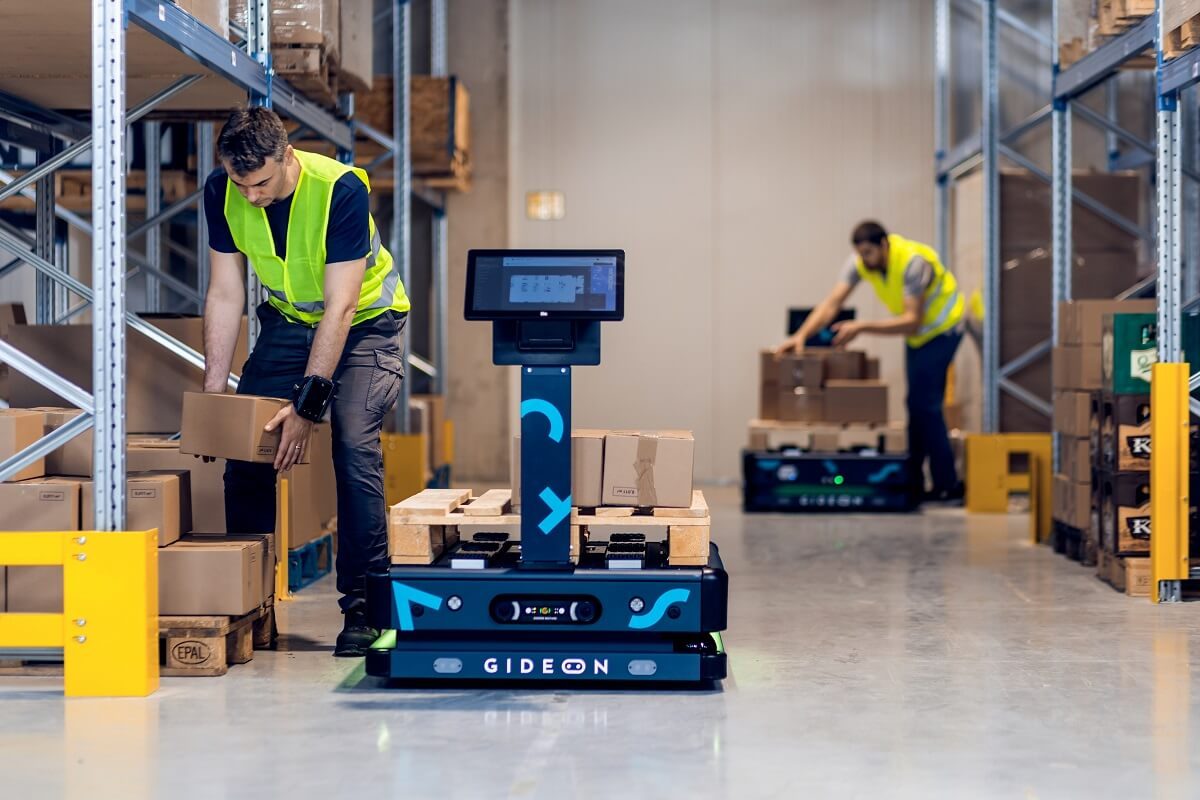
- Optimizing Operations
- blog
Item picking vs. case picking order fulfillment
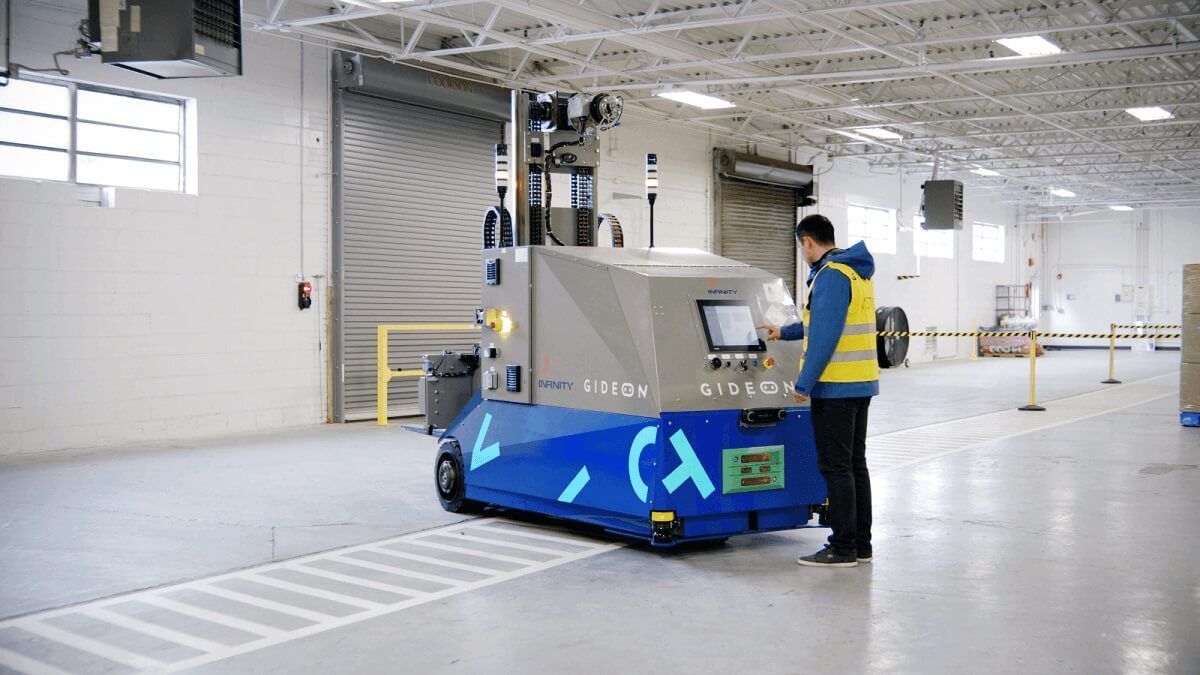
- blog
The advantages of autonomous forklifts
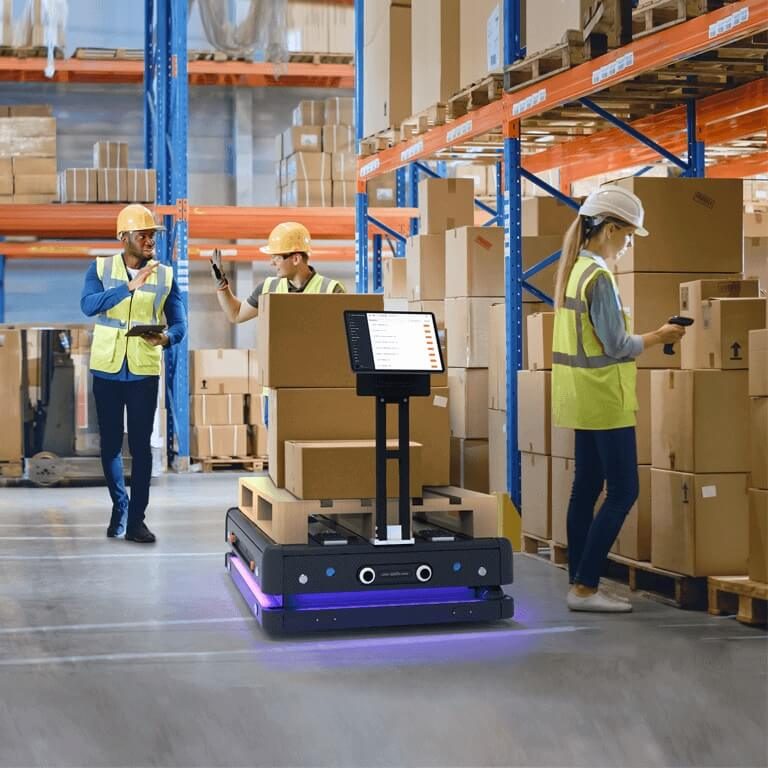
- blog
The pros and cons of Autonomous Mobile Robots
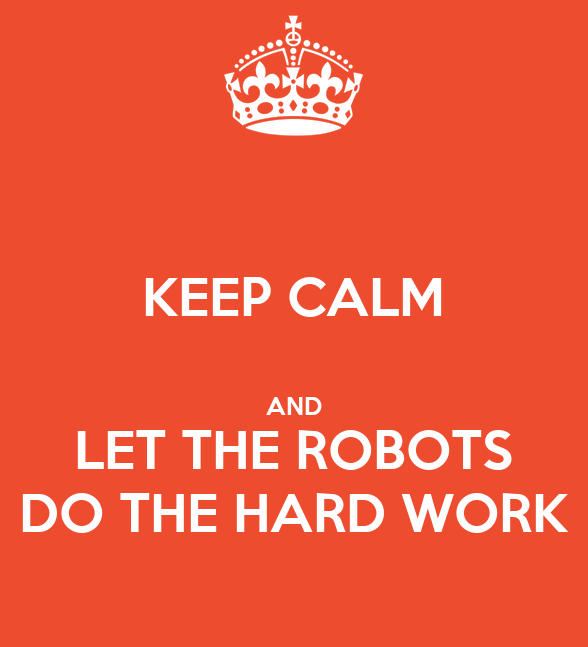
- blog
The Gideon visual SLAM pipeline for the warehouse of the future
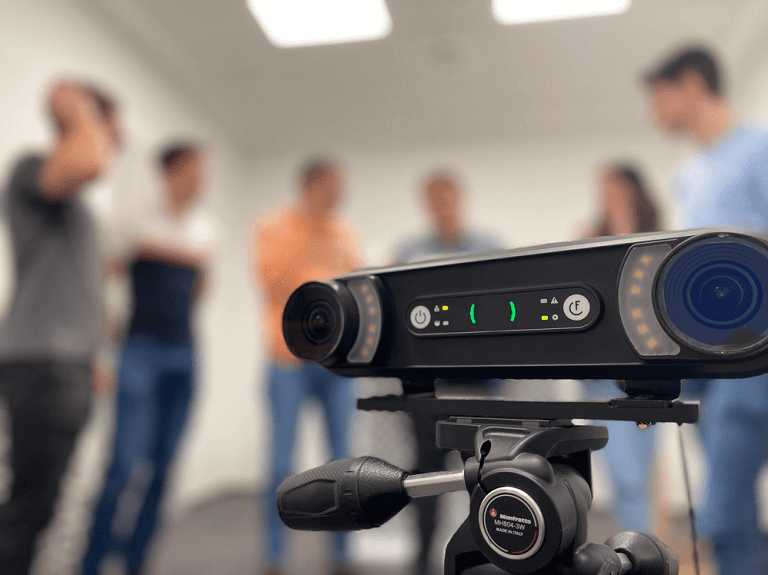
- blog
3D depth sensing for robots 101
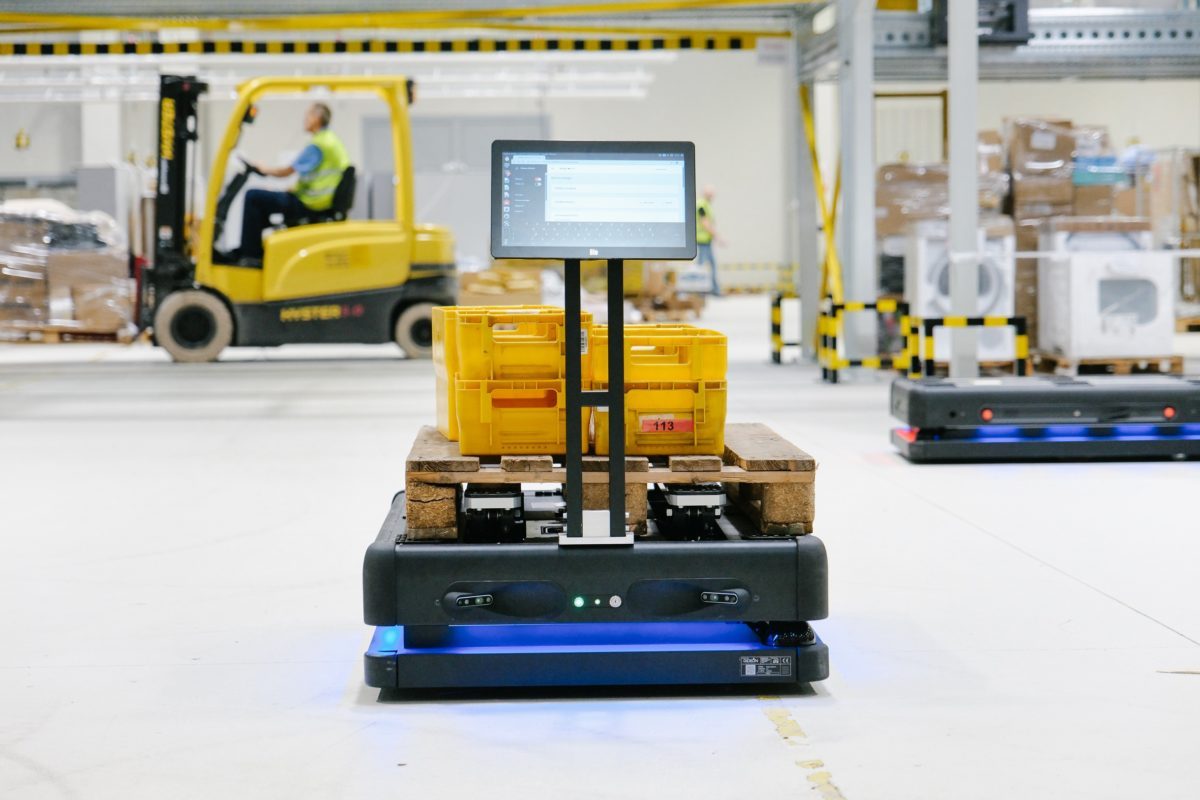
- Optimizing Operations
- blog
Seven signs you need (more) warehouse automation
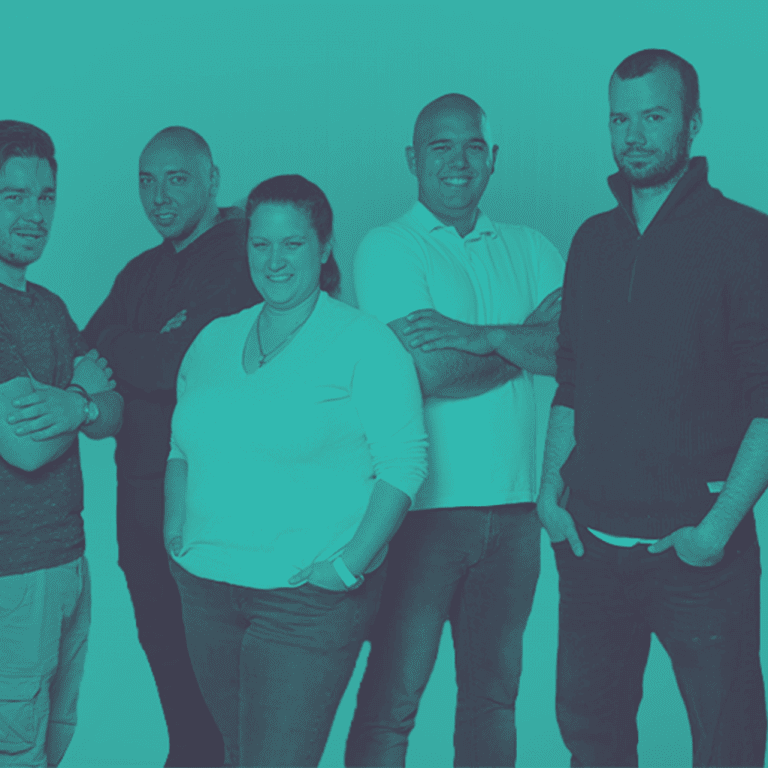
- blog
Everything starts with people
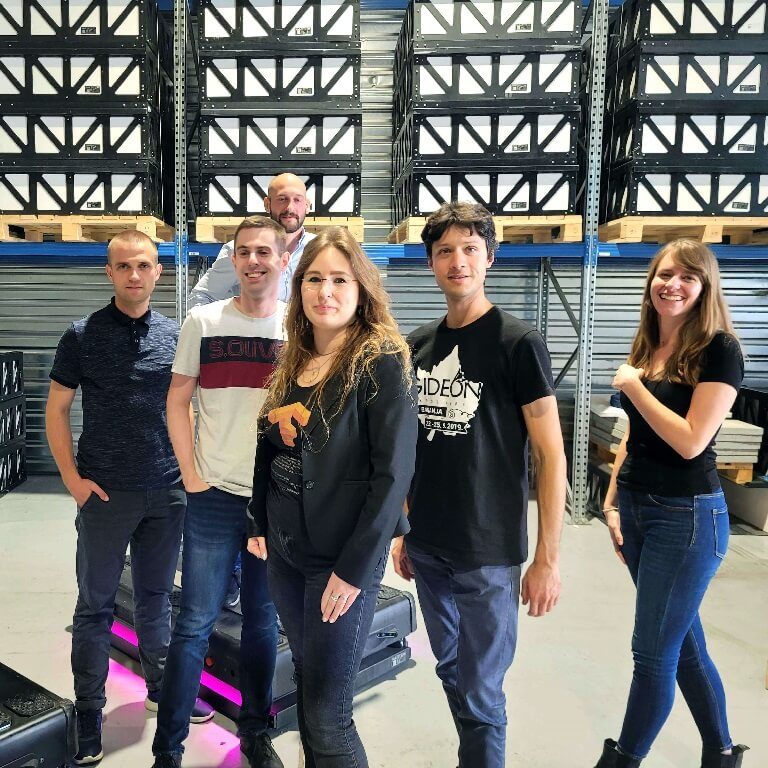
- blog
PyTorch at Gideon in 2021: A year in review
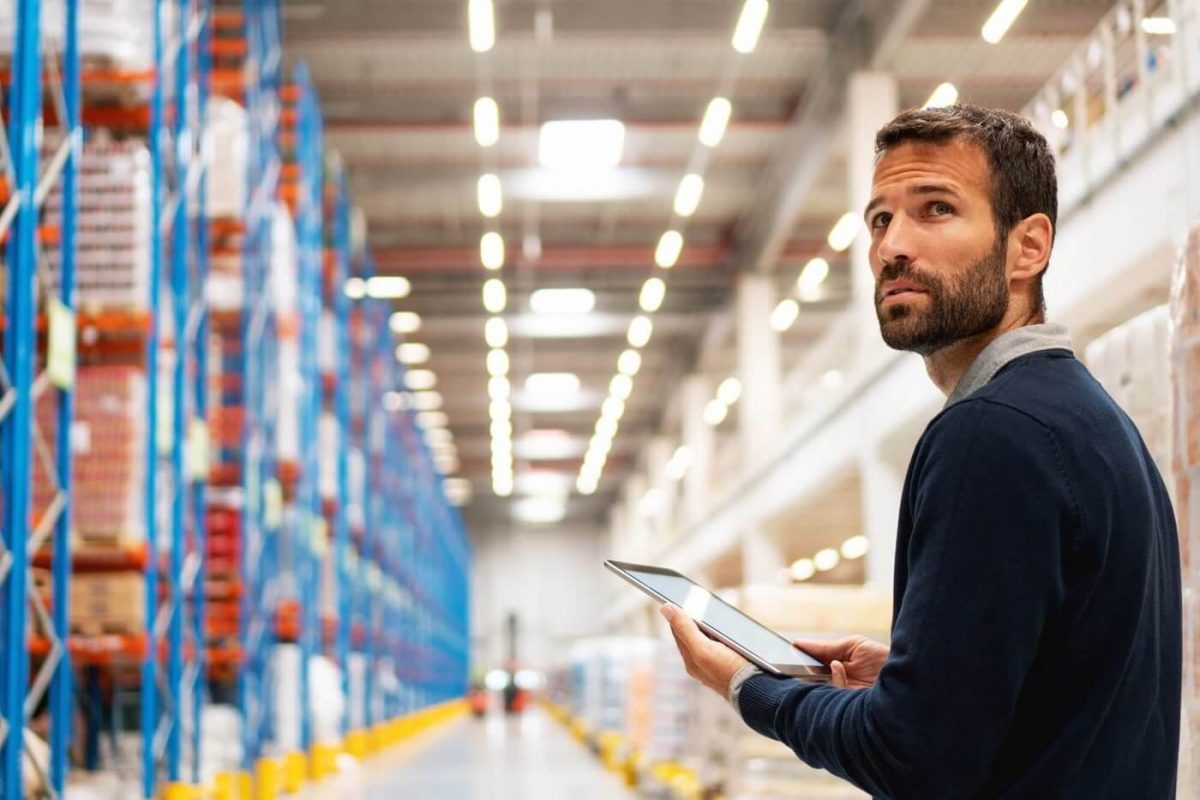
- Solving Labor Challenges
- blog
How to solve the long-term warehouse labor shortage